Today, the AI landscape seems to be experiencing its most dramatic growth yet, a phenomenon that is both intriguing and perplexing given that the field has been developing for nearly 70 years.
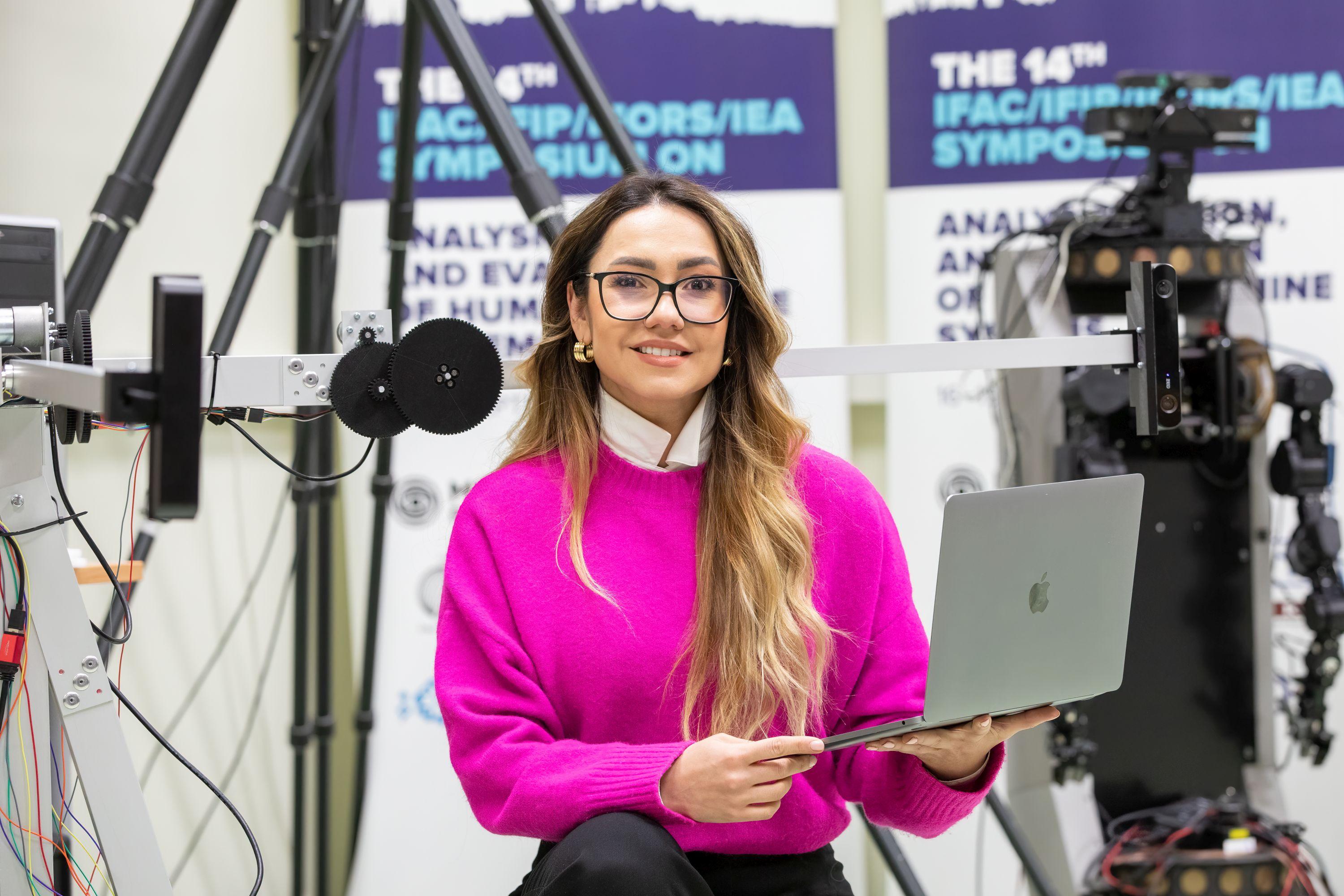
Elli Valla | Photo: Karl-Kristjan Nigesen
Back in the 1950s, Norbert Wiener, a mathematician and pioneer of cybernetics, issued early warnings about the existential risks posed by machines. He even revisited fears from the 1860s1 that machines would eventually out-evolve and dominate humans. In contrast, IBM’s computer scientist Arthur L. Samuel, who developed a groundbreaking self-learning chess-playing program2 in the 50’s, asserted that machines are merely tools without will or magic. Considering that the dystopian predictions of the 1950s have not materialized, the question arises: is the current situation truly different, or is this just the next chapter in AI's long history?
By the 1980s, the computer revolution had taken hold, triggering a form of 'computerphobia'3 that raised concerns about machine displacement of human jobs and ensuing societal upheaval. Fast forward four decades, and these discussions have resurfaced amid continued AI advancements, reigniting debates about job loss and ethical considerations.
A pivotal event occurred in 2012 when deep learning models excelled in Stanford's ImageNet4 competition, a benchmark for computer vision. This leap wasn't solely due to powerful Graphics Processing Units (GPUs); it was also fueled by vast datasets and the advent of Convolutional Neural Networks (CNNs). These developments have revolutionized several industries, including healthcare, where enhanced image recognition now facilitates the highly accurate interpretation of MRIs, X-rays, and other diagnostic scans.
Another transformative development took place in 2017. Google Brain's team published a paper titled "Attention Is All You Need,"5 introducing a new generation of neural network architectures called Transformers. Since then, these have become the foundation for modern Natural Language Processing (NLP), serving as the basis for well-known language models like BERT and the GPT series, where the "T" stands for Transformer.
One reason the AI field seems to be in its most dynamic phase of growth is the democratization of AI. Powerful language models such as ChatGPT, Bard, Claude, and others have lowered the entry barriers, allowing people without a technical background to interact with complex AI systems. This marks a departure from earlier stages of AI development, which were mainly the realm of computer scientists and specialized engineers.
The Diagnostic Power of Handwriting Kinematics
As a PhD student and early-stage researcher at TalTech University's Department of Software Science, my work shines a spotlight on a niche but impactful area where AI is revolutionizing healthcare: human motor function analysis. At the heart of our research are machine learning algorithms designed to decode complex human motor skills. One focus is on using tablet PCs and AI to analyze the handwriting patterns of individuals with Parkinson's disease6. Handwriting serves as a neurological window, offering rich diagnostic data from the kinematics of motion, such as velocity and trajectory angles. By utilizing AI algorithms, we can detect subtle abnormalities that may act as early indicators of the disease.
However, our research scope extends beyond Parkinson's. The analytic principles used in handwriting studies are equally applicable to fatigue assessments7, facilitated by smartphone sensors that capture fine motor signals, markers for cognitive decline. The incorporation of smart devices into our methodology heralds a new era of remote diagnostics, significantly benefiting those with mobility challenges and reducing the strain on healthcare systems.
Our work represents merely a fraction of the transformative potential that AI holds. As we proceed further into the 21st century, it's clear that AI will continue to enhance the accessibility, efficiency, and effectiveness of healthcare.
Language Models: The Digital Assistants Revolutionizing Medical Practice
The potential of Large Language Models (LLMs) in the healthcare sector is significant, especially in areas such as medical diagnosis, treatment recommendations, and administrative tasks. LLMs can substantially accelerate healthcare workflows through automating routine activities, such as sorting medical data, drafting preliminary reports, and answering standard patient questions. This efficiency allows healthcare professionals to focus on more complex aspects of patient care that current AI is not yet capable of handling. Furthermore, being freed from routine tasks enables healthcare workers to dedicate time to continuous learning and professional development, advancing the limits of modern medicine.
In this context, Google's LLM named MedPaLM28 is a noteworthy milestone. This language model has been fine-tuned with a massive dataset of medical questions and answers and was the first to achieve an "expert" level in medical licensing exam questions. MedPaLM2's ability to generate and understand medically relevant text can significantly contribute to diagnosis, treatment planning, and patient education, making it a potentially transformative change in healthcare technology.
Looking forward, the concept of "digital twins"—a complete digital replica of an individual's health data—is among the most transformative visions. Imagine physicians running simulations on a digital twin before implementing treatments on the actual patient, thus minimizing risks and tailoring treatment plans more precisely. The ability to adjust treatments in real-time based on simulated outcomes could revolutionize the treatment process.
The Cautious Optimism: Balancing AI's Promise and Peril
While the transformative potential of AI is abundantly clear, it's also crucial to be mindful of its risks. Personally, I'm inclined toward an optimistic viewpoint. Many in the field are already discussing heavy regulation or even halting development due to the far-off concern of Artificial General Intelligence (AGI). However, AGI is not here yet, and there are more immediate, manageable risks that we should focus on.
The real dangers lie in the more direct and current risks like AI-generated misinformation or hallucinations9, biases in training data, and the threat of identity theft through deep fakes. These issues could lead to incorrect medical diagnoses, discrimination, or unauthorized access to sensitive information, respectively. Therefore, it's important to understand that AI-generated outcomes should not be considered 100% reliable. From another perspective, AI models can be trained to detect and label harmful information, acting as a countermeasure to these challenges.
There's also a growing fear that AI could lead to mass job displacement, rendering many traditional roles obsolete. However, it's essential to recognize that AI also creates new job opportunities that didn't exist before. For instance, the rise of AI has led to an increased demand for data labelers, who annotate and clean data to train machine learning models. Similarly, AI-powered chatbots require human "trainers" to help fine-tune their algorithms for more accurate and nuanced responses. These are jobs that often don't require specialized technical skills, making it easier for people to transition into these roles.
Innovation Before Regulation
While these risks merit serious attention, I believe that innovation should precede regulation. Preemptive and potentially harmful restrictions could stifle AI’s progress. By fostering responsible development, we can better understand and subsequently mitigate these risks. This approach not only refines the technology but also informs the creation of balanced, not stifling, regulations.
In conclusion, AI stands on the brink of becoming one of humanity's most invaluable allies, unlocking advancements once confined to the realm of imagination.
1 https://newsletter.pessimistsarchive.org/p/the-original-ai-doomer-dr-norbert
2 https://www.chessprogramming.org/Arthur_Samuel
3 https://www.theatlantic.com/technology/archive/2015/03/when-people-feared-computers/388919/
4 https://www.image-net.org/challenges/LSVRC/
5 Vaswani, Ashish, et al. „Attention is all you need.“ Advances in neural information processing systems 30 (2017).
6 Valla, Elli, et al. „Tremor-related feature engineering for machine learning based Parkinson’s disease diagnostics.“ Biomedical Signal Processing and Control 75 (2022): 103551.
7 Valla, Elli, et al. „Transforming fatigue assessment: smartphone-based system with digitized motor skill tests.“ International journal of medical informatics 177 (2023): 105152.
8 http://sites.research.google/med-palm
9 The term refers to instances where an artificial intelligence system generates outputs that appear plausible but are in fact entirely fabricated, false, or based on misconceptions. These outputs can mislead users or systems that rely on accurate data, posing potential risks in various applications.