Over the past few years, a number of new Explainable Artificial Intelligence (XAI) techniques have been developed to improve the explainability of machine learning models. One such research article that analyses the above developments was recently published in the journal Energy & AI.
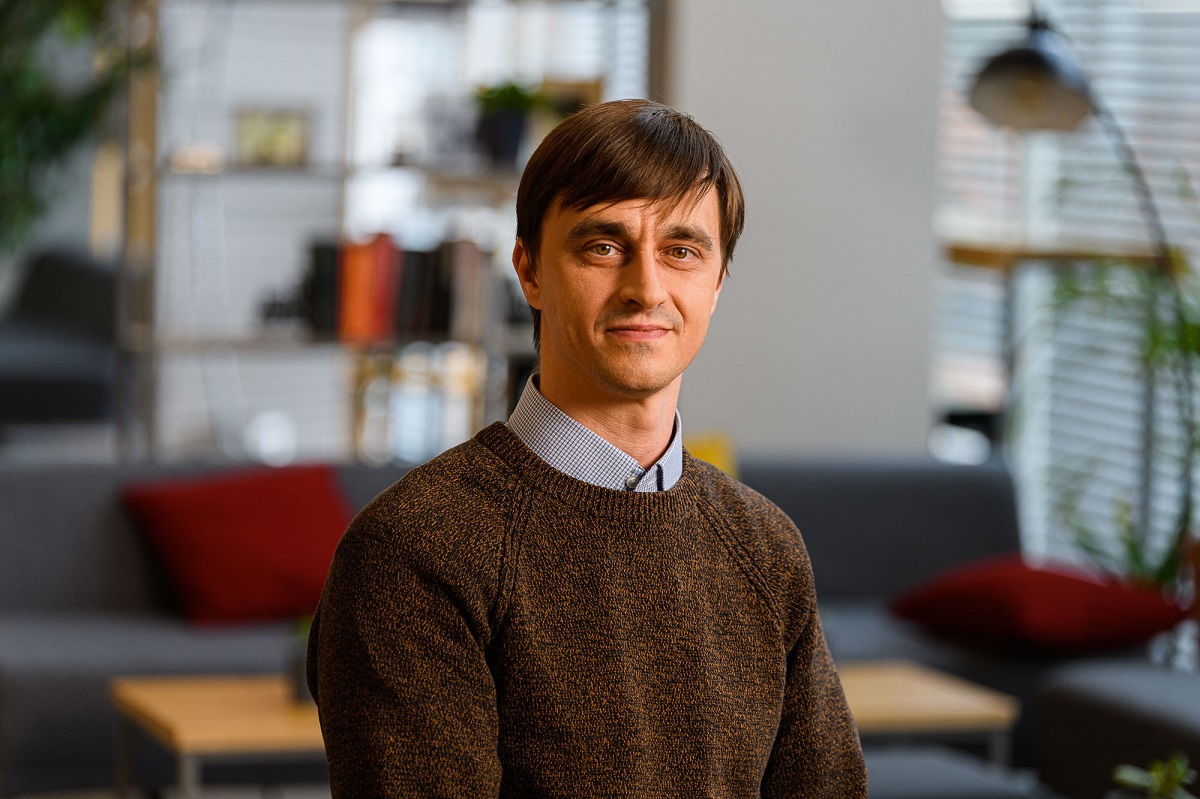
One of the authors of the article entitled ‘Explainable Artificial Intelligence (XAI) techniques for energy and power systems: Review, challenges and opportunities’ is Juri Belikov, Tenured Assistant Professor at the TalTech School of Information Technologies, who explained that in the article their main focus was on the use potential of XAI in energy and power systems. Their research also addresses the major challenges and limitations in this field.
‘The article also includes an overview of the latest research on the topic of XAI in the energy domain and an analysis of the current trends,’ Belikov notes, adding that it is his hope that this article launches fruitful discussions and encourages researchers to keep working on this complex topic which is of vital importance for our future. The development of AI benefits several sectors and groups, including research and science, industry, and all electricity consumers!
Deep learning helps us advance to the next level
The objective of XAI is to help researchers, developers, domain experts, and users to better understand the inner operation of machine learning (ML) models. Despite widespread adoption and outstanding performance, machine learning models are still considered to be ‘black boxes’, as it is very difficult to understand how such models operate in practice. Therefore, in the power systems field, which requires a high level of accountability, it is hard for experts to trust and justify the decisions and recommendations made by these models.
Thanks to the evolution of deep learning (DL), better classifiers and machine learning algorithms have been developed for power system applications in the last few years. In certain scenarios, these deep learning techniques seem to have advantages compared to traditional algorithms, for example, in terms of efficiency, noise immunity, and accuracy.
But what are the main limitations of XAI in the energy and power systems domain? Despite the evident success of such algorithms, an inherent difficulty is that machine-learning models are often very complex, as a result, it may not be clear how or why they make certain decisions, and how they treat real-world data.
For instance, the planning and operation of power systems is carried out solely by power experts, who rely on their knowledge which they have gained over time in the field of power systems, supporting programs, and as part of field experience. Therefore, experts in the power system field may find it hard to trust the decisions and recommendations made by machine-learning-based algorithms, which limits their practical use.
This difficulty is especially prominent in cases that require a high level of reliability (which is common in the energy industry). Due to this challenge, new techniques and principles have been developed in the last couple of years to improve the explainability of machine learning models, so that their output can be better understood.
While the article is valuable in that it highlights the potential of using XAI for energy and power systems applications, also covering the challenges and limitations faced, the review of recent works on the topic of XAI in the energy domain presented in the article is equally important. Among other things, the authors reveal which XAI techniques are the most popular and why, and explain why specific methods are used for these applications.
In conclusion, while XAI can facilitate the use of ML techniques in practice, there are still a number of obstacles that should be taken into consideration, such as standardisation, security, and incorrect confidence. In the article, potential applications and future research directions related to XAI and energy are also presented (e.g. optimal energy management and control, energy consumer applications, and power system monitoring).
According to the authors, one future line of research with high potential is the use of XAI for faster integration of renewable energy sources and energy storage units in various applications. Another recommended direction is to focus even more on DL models as, despite their high accuracy, they still cannot be trusted to the extent necessary.
You can read the research article in the latest issue of Energy & AI.