Global urbanisation is causing many problems, including high levels of urban noise, traffic congestion, and greenhouse gases. It represents a significant source of costs for cities and has a negative impact on our living environment.
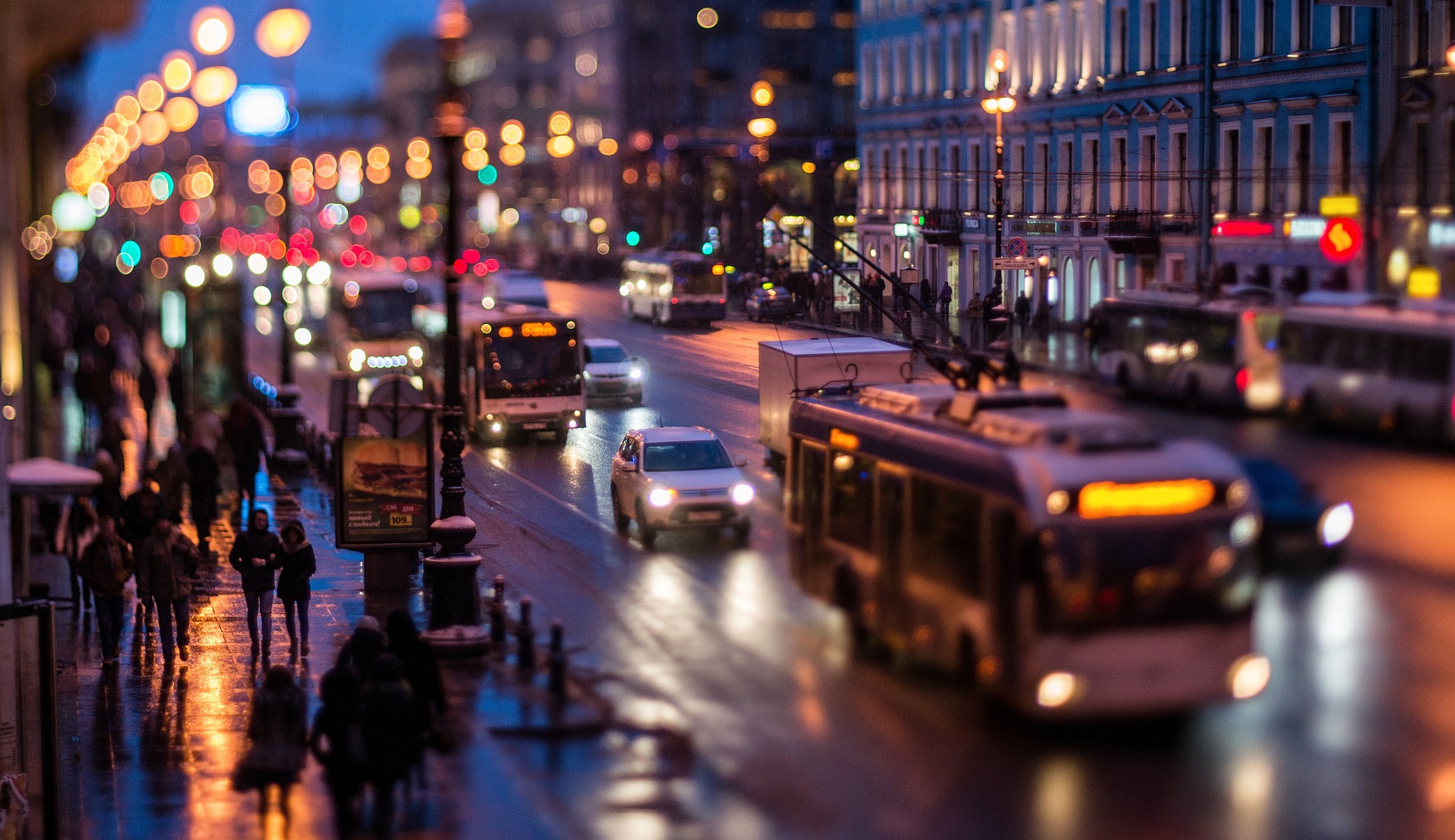
Andres Suislepp is a long-time resident of Tallinn, he has occasionally travelled from point A to point B in the capital on foot, has occasionally used a bicycle, and sometimes even a car to reach his destination. While using different modes of transport, he increasingly found himself thinking that our urban environment could be more convenient and at the same time more sustainable.
Suislepp knew that large amounts of data on our movements are being collected to tackle the problems caused by urbanisation, but working with specialised big data of this kind is complex and expensive, and requires a lot of computing power.
So that he wouldn’t have to sit around waiting around for changes to happen, Suislepp chose the topic ‘Multi-modal data fusion for short-term urban noise prediction in intelligent transportation systems’ for his Master’s thesis at TalTech's School of IT.
The goal set by Suislepp was that the outcome of the work could be used to further develop intelligent transportation systems. In addition, the solution he created can be used to help build applications that would also help make life more convenient for city residents.
The prediction model comes to the rescue
Intelligent transportation systems are a set of applications and services based on modern information and communication technologies that aim to make transportation systems more efficient, safer, and more sustainable for society and the environment.
Suislepp’s Master’s thesis proposes a hybrid data fusion strategy to better tackle the problems of urban noise, traffic congestion, and CO2 emissions – a model for urban noise prediction based on data from the City of Tallinn was completed.
When validating the model’s results it turned out that the model works very well for short-term forecasts, achieving an average error rate of less than three per cent. At the same time, the data fusion strategy simplified the model itself, leading to nearly three times less computing power being used when compared to before.
‘The predictions made by the developed model can be used to build applications for end-users that would help make our urban spaces safer and more convenient,’ explains Suislepp.
Surprising efficiency
Suislepp points out that one of the things that surprised him during the course of his Master’s thesis was that the use of simple statistical algorithms proved to be quite effective and in the case of data fusion increased both the accuracy of the models while at the same time also simplifying the model.
‘At a time when energy prices are high and computational resources require a great deal of power, the simplicity of the models carries a lot of weight,’ says Suislepp.
According to Suislepp, the most important result of his Master’s thesis is the model for urban noise prediction developed in the course of research, which showed a very good level of accuracy in the case of short-term, 5- and 15-minute predictions. The model could be generalised to other traffic characteristics, based on which a number of useful applications for citizens could be built.
As the main focus of the work was on data fusion, the simplicity and low resource requirements of the developed models give it an advantage for implementation in real-life applications, as it is inexpensive to use compared to conventional machine learning applications.
Making life more convenient
Suislepp says that although the solution he has created is purely technical, it is possible to build applications based on it, which would help make life more convenient for end users, or city residents.
For example, it could be used as the basis for creating systems that would inform people about areas where the urban noise level may reach a very high level in the short term and which should be avoided at certain periods of time.
In addition, urban noise is highly correlated with the density of road traffic, and urban noise predictions could be used to build, for example, adaptive traffic light systems that would improve the capacity of our streets.